Artificial intelligence, or can machines think?
May 3, 2018·Alasdair MacleodArtificial intelligence (AI) is seen as both a boon and a threat. It uses our personal data to influence our lives without us realising it. It is used by social media to draw our attention to things we are interested in buying, and by our tablets and computers to predict what we want to type (good). It facilitates targeting of voters to influence elections (bad, particularly if your side loses).
Perhaps the truth or otherwise of allegations such as electoral interference should be regarded in the light of the interests of their promotors. Politicians are always ready to accuse an opponent of being unscrupulous in his methods, including the use of AI to promote fake news, or influencing targeted voters in other ways. A cynic might argue that the political class wishes to retain control over propaganda by manipulating the traditional media he understands and is frightened AI will introduce black arts to his disadvantage. Whatever the influences behind the debate, there is no doubt that AI is propelling us into a new world, and we must learn to embrace it whether we like it or not.
To discuss it rationally, we should first define AI. Here is one definition sourced through a Google search (itself the result of AI):
“The theory and development of computer systems able to perform tasks normally requiring human intelligence, such as visual perception, speech recognition, decision-making, and translation between languages.”
This description is laced only with the potential benefits to us as individuals, giving us facilities we surely all desire. It offers us more efficient use of our time, increasing productivity. But another definition, which might ring alarm bells, is Merriam-Webster’s: “A branch of computer science dealing with the simulation of intelligent behaviour in computers. The capability of a machine to imitate intelligent human behaviour.”
Now we are imitating humans, particularly when we add in the ability of machines to learn and adapt themselves to new stimuli. Surely, this means machines are taking over jobs and even our ability to command. These are sensitive aspects of the debate over AI, and even the House of Lords has set up a select committee to report on it, which it did last week.[i] Other serious issues were also raised, such as who do we hold accountable for the development of algorithms, and the quality of the data being input.
This article is an attempt to put AI in perspective. It starts with a brief history, examines its capabilities and potential, and finally addresses the ultimate danger of AI according to its critics: the ability of AI and machine learning to replicate the human brain and thereby control us.
AI basics
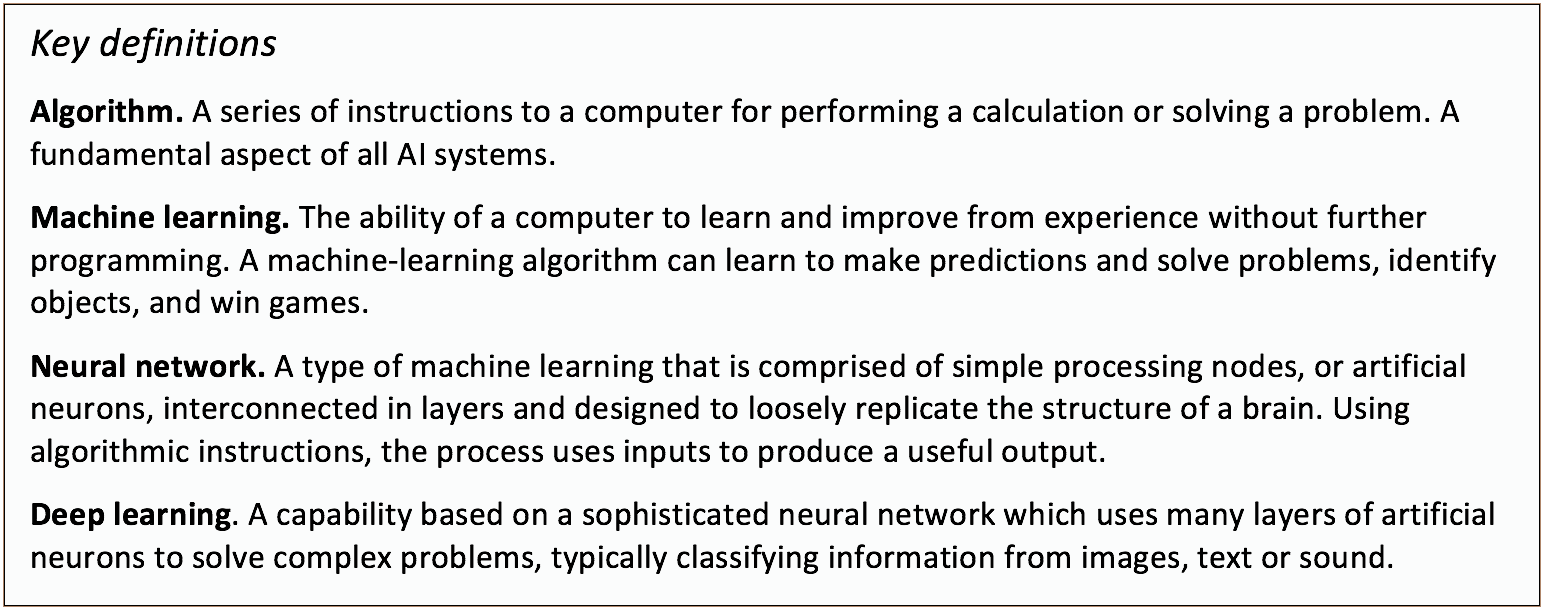
AI has always been an integral part of computer development. As long ago as 1950, Alan Turing published a paper, Computing Machinery and Intelligence, which posed the question, “Can machines think?”.[i] It was the concept of a “Turing Test” that determined whether a machine has achieved true AI, and the term AI itself originated from this period. The following decade saw the establishment of major academic centres for AI in the US at MIT, Carnegie Mellon University, Stanford, and Edinburgh University in the UK.
The 1980s saw governments become involved, with Japan’s Fifth Generation project, followed by the UK Government launching the Alvey Programme to improve the competitiveness of UK information technology. This effort failed in its central objective, and the sheer complexity of programming for ever-increasing rule complexity led to a loss of government enthusiasm for funding AI development. In the US, the Defence Advanced Research Projects Agency also cut its spending on AI by one third.
However, in the late-1980s, the private sector began to develop AI for applications in stock market forecasting, data mining, and visual processing systems such as number plate recognition in traffic cameras. The neural method of filtering inputs through layers of processing nodes was developed to look for statistical and other patterns.
It was only since the turn of the century that the general public has become increasingly familiar with the term AI, following developments in deep learning using neural networks. More recently, deep learning, for example used for speech and image recognition, has been boosted by a combination of the growing availability of data to train systems, increasing processing power, and the development of more sophisticated algorithms. Cloud platforms now allow users to deploy AI without investing in extra hardware. And open-source development platforms have further lowered barriers to entry.
While the progress of AI since Turing’s original paper has been somewhat uneven, these new factors appear to promise an accelerating development of AI capabilities and applications in future. The implications for automation, the way we work, and the replacement of many human functions have raised concerns that appear to offset the benefits. There are also consequences for governments who fail to grasp the importance of this revolution and through public policy seek to restrict its potential. Then there is the question of data use and data ownership. I shall briefly address these issues before tackling the philosophical question as to whether AI and machine learning can ultimately pass the Turing test in the general sense.
The work-AI balance
AI and machine learning are already with us in more ways than most of us are aware. A modern motor car can now drive itself with minimal human input, and these abilities are no longer just experimental, becoming increasingly common on all cars. Navigation systems use AI to determine route choices in the light of current traffic congestion. Border controls use iris recognition to match passport data with the person. Airlines price their seating dynamically, and AI is increasingly used for health diagnosis, detecting early signs of cancer being one example. Hidden from us, businesses are increasingly using AI embedded in their internal systems to deliver services more efficiently. AI and machine learning are rapidly becoming ubiquitous.
These applications are all narrow in scope, in the sense they perform specific tasks that would require intelligence in a human. They involve one or two categories of data and often both, depending on the application. The first type is general data, such as that used to generate weather and price forecasts, the second being personal to individuals.
Privacy laws for personal data are becoming more and more restrictive, as governments clamp down on their use. The more aggressively governments do this, the less flexibility a business has in devising AI solutions for those whose data they use. Therefore, a government which takes account of both personal privacy issues and the benefits of narrow-scope AI is likely to see more economic advancement in this field than one that fails to make the distinction. The British and American governments appear to be friendlier to the leaders in the field in this respect than the EU. The EU has fined nearly all the big US tech companies, often on questionable grounds, hardly displaying a constructive attitude to future technological development. And while there are pockets of entrepreneurship in Continental Europe, they come nowhere near competing with the twin nexus of London and Oxford.
This may be important, post Brexit. Last month, the UK Government announced a £1bn investment programme with the ambition to make Britain the best place to start and grow an AI business. Meanwhile, the EU’s attitude is broadly parochial, protectionist, anti-change and appears to be particularly antagonistic against the large American corporations that have done so much to advance AI.
AI is becoming critical for job creation. Every technological change in recorded history has been condemned in advance as destroying jobs, and every technological change has ended up creating them instead and improving both the quality of life and earnings of the average person. There’s no reason to think that AI will be any different. It is that unmeasurable thing, called progress, that ensures jobs are created, and job creation is always the result of positive change.
Like nearly every other invention deployed for the ultimate benefit of the consumer, AI is a creature of the private sector, not government. It is never the function of a democratic government to innovate, so it must resist the temptation to prevent change arising from the private sector, particularly when it comes to protecting jobs. Furthermore, unnecessary regulation serves to hand progress in AI on a plate to other jurisdictions, such as China, whose government is semi-entrepreneurial and is aggressively developing and deploying AI for its own national interest.
Those who have a limited grasp of free markets fail to understand that AI, in the narrow sense we are addressing here, will produce its own solutions to concerns over data and automation. For example, Sir Tim Berners-Lee[ii] is currently developing with MIT a project to result in “true data ownership as well as improved privacy”, which if successful will not only make recent privacy regulation redundant, but also address the use of personal data by unscrupulous operators exploiting loopholes in the regulations, and ensure the jurisdictional limits of the law can no longer be exploited.[iii]
The balance between work and AI, so long as governments take a minimalist approach to regulation, promises to be enormously beneficial to ordinary people, improving life-quality, much as the data revolution through the internet has done over the last twenty-five years. We cannot forecast the precise outcome, because the development of new technologies is always progressive in nature, with one step leading to others not yet in view. However, the limitation of AI so far has been its application is always narrow in context, for the ultimate benefit of consumers. The application of AI in the general sense, where computers and robots acquire the ability to control humans in a nightmarish brave new world is perhaps what frightens the uninformed, with comments such as, “where will it all end?” This is our next topic.
General AI
All AI has been developed for applications in the narrow sense, in other words to perform specific tasks intelligently. By intelligently, we refer to machine learning and deep learning applied in a specific context only. A computer can now beat a chess grandmaster, and in a more recent achievement DeepMind, a UK-based Google subsidiary, mastered the game of Go.
These achievements suggest that AI is now superior to the human mind, but this is only true for defined tasks. So far, little or no progress has been made in AI for non-specific, or general applications. This could be partly because there is little demand for a machine with non-specific applications, or the data and processing required is uneconomically substantial. Perhaps it is a question for the next generation of AI, which might attempt the algorithms. However, the practical hurdles are one thing, and whether it can ever be achieved is essentially a philosophical question.
There is a clash of sciences involved, between the world of physics, which works to rules, formulae and laws, and human nature, which is only guided by them. Machine and deep learning relies on continual updating from historic data to detect patterns of human behaviour from which outcomes can be forecast. The fact that machines have to continually learn from new inputs tells us that AI is in a sense a misnomer: Alan Turing’s test, can machines think, is and always should be answered this way: they only appear to think.
This is a vital distinction. Yes, we all use data and experience to help form our decisions, and yes, deep learning allows a machine to always process that information better than a human. But where the two diverge is over choice. A machine must always choose a true/false output from all its inputs. A human always has a choice, which when taken may appear irrational to an observer. And we can then ask the question, is it the chooser or the observer who is irrational? To make this point another way, if the machine makes a choice that a human does not accept, the human can ignore it, use it as a basis for making an entirely different choice, or even switch the machine off.
This is the fundamental difference between machine learning and human thought processes. A machine produces outputs that are essentially binary: act or not act, turn inputs into an image which is recognised or not recognised, and so on. A human can be logical, illogical or even a combination of the two, and is rarely tied to binary outcomes.
For these reasons we can be confident that machines will never take us over, in the sense that is often predicted in science fiction. But this still leaves the unanswered question as to why is it that algorithms are so successful in trading financial instruments, which involves forecasting the human action that sets tomorrows prices. Far from being proof of a machine’s intellectual superiority, it provides a good example of the difference between human intelligence and AI, and the latter’s limitations.
Computer, or algorithmic trading comes in two different objectives in mind. There is the trading that involves dealing at a human’s behest, such as rebalancing an index-tracker, or reallocating ETF inflows and outflows to and from investments is accordance with the objective. We will put this mechanical function to one sides. Alternatively, it is used for automated trading for profit, which is where the controversy lies.
Automated trading is not intelligent in the human sense, being based on rules applied to historic data, continually modified as new data is added. It assumes that past trading patterns will be repeated, and then through the magic of electronics beats slower human thinkers and rival machines by placing orders and having them executed in milliseconds. Humans trading involves their experience, pattern recognition, factors external to the price such as news, innate ability and emotion. The combination of these factors makes human performance both successful and unsuccessful and introduces intuition. The strength and success of computer trading is down to the lack of human factors in securities trading, not because it replicates them.
The increasing presence of computer trading in markets probably extends cyclical trends further into overvaluation and undervaluation territories than would otherwise be the case. This statement is conditioned by the lack of firm counter-factual evidence to prove it, but if a significant portion of total trading in a particular instrument is conducted purely on an extension of past trends, this seems likely. In other words, AI ends up driving earlier AI-driven prices, reducing the human element in pricing instead of forecasting it.
The wider implications of these distortions are beyond the scope of this article, which is to debate whether AI is a boon or a threat. We have established that AI and machine learning is and will be an enormous assistance to mankind, and that the fears of a brave new world where machines are the masters and humans the slaves is incompatible with science. Fears over job losses from AI, in common with fears over job security with all pervious technological developments, are misplaced.
And if Alan Turin were alive today, it would be interesting to know if his question, can machines think, has been answered to his satisfaction. The evidence is it has not and perhaps never will be.
[i] This article draws on the House of Lords report for some of its information. See https://publications.parliament.uk/pa/ld201719/ldselect/ldai/100/100.pdf
[ii] https://home.manhattan.edu/~tina.tian/CMPT420/Turing.pdf
[iii] Credited with the initial development of the world-wide web.
[iv] See https://solid.mit.edu/
The views and opinions expressed in this article are those of the author(s) and do not reflect those of Goldmoney, unless expressly stated. The article is for general information purposes only and does not constitute either Goldmoney or the author(s) providing you with legal, financial, tax, investment, or accounting advice. You should not act or rely on any information contained in the article without first seeking independent professional advice. Care has been taken to ensure that the information in the article is reliable; however, Goldmoney does not represent that it is accurate, complete, up-to-date and/or to be taken as an indication of future results and it should not be relied upon as such. Goldmoney will not be held responsible for any claim, loss, damage, or inconvenience caused as a result of any information or opinion contained in this article and any action taken as a result of the opinions and information contained in this article is at your own risk.